3D imaging, especially LiDAR (Light detection and ranging) technique, is gaining increasing importance in many emerging autonomous applications such as self-driving cars, indoor robots, drones, and in advanced scientific, medical, and industrial applications. The superior properties of light-waves in LiDAR provide an ideal choice for 3D imaging, offering fine volumetric resolution and long operational distance. However, current LiDAR solutions suffer from serious challenges, including high power consumption, bulky size, high cost and poor performance. Therefore, researchers are pushing toward developing advanced LiDAR solutions with satisfying performance and reasonable power budget, size, and cost.
The most popular LiDAR architectures are the amplitude-modulated continuous-wave (AMCW), frequency-modulated continuous-wave (FMCW) and pulsed LiDAR. In our research at ISL, we focus on the pulsed LiDAR architecture using Single-photon avalanche diodes (SPAD) to measure time-of-flight (ToF) employing CMOS time-to-digital Converters (TDCs) for compact, low-cost, and highly integrated 3D sensing. The TDC specifications are critical determinants of the system’s range, resolution, and accuracy. We focus on modelling and optimizing the TDCs, SPADs, and the 3D sensing system to capture the target application’s design specifications and parameters under different operating scenarios.
More specifically, time-correlated single-photon counting (TCSPC) 3D imaging requires the digitization of photon arrival times across an array of SPADs (Figure 1). The most critical performance metrics of TDCs in TCSPC applications are their conversion rate (CR), area, and power consumption. In our research we present a multi-channel RO-based TDC architecture whose power consumption scales with its configurable resolution for power-sensitive applications [1]. Further power savings are achieved by sharing one RO among multiple TDC channels. We demonstrate that sharing one RO among five channels reduces power consumption by more than 75% relative to non-shared architectures.

A 5-channel 12-bit TDC is fabricated in 65 nm CMOS with an area of 1920 µm2 per channel. It demonstrates CR up to 125 MHz and offers a resolution configurable over the range of 24–133 ps. Figure 2(a) shows the proposed configurable arrayble RO-based TDC structure for SPAD-based TCSPC applications. This design uses an asynchronous counter consisting of a cascade of toggle flip-flops (TFF) as frequency dividers (FD). Figure 2(b) shows a microphotograph of the proposed 5-channel TDC fabricated in 65 GP CMOS technology. The chip occupies an active area of 120 μm x 80 μm, excluding the serializers and output buffers.
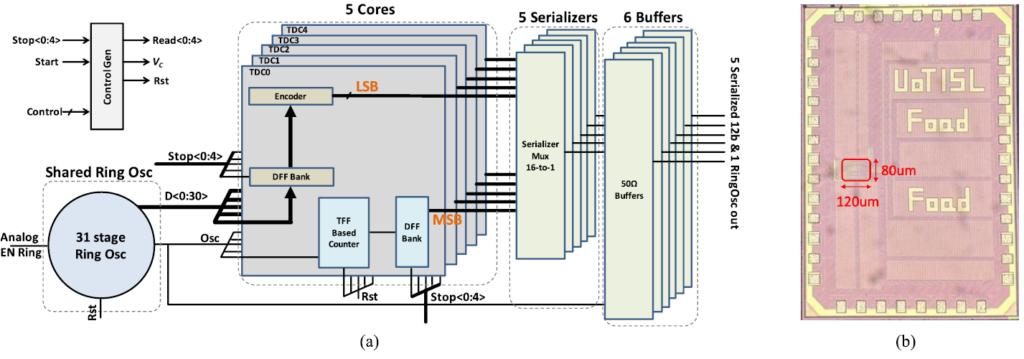
As the single-photon detection is fundamentally a stochastic process, we develop a fast and accurate analytical system model relates the number of required TDCs per SPAD array to the 3D imager performance accounting for environmental factors such as ambient light and distance [2]. Our system model permits architectural exploration to determine the number of TDCs required for a given array size. It also reveals that the most critical performance metrics of TDCs in time-correlated single-photon counting (TCSPC) applications are their CR, area, and power consumption. Monte Carlo numerical simulations verify the accuracy of our method. Figure 3 confirms that increasing the number of TDCs beyond 15 has no significant effect since very few arrivals would be time-stamped by TDCs #16 onward.
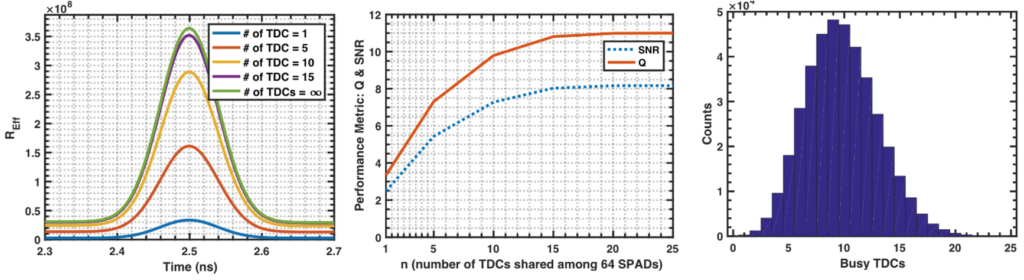
In addition, we study the jitter of RO-based TDCs as a function of their full-scale range, resulting in an expression for the TDC total jitter [3]. The analysis also identifies a peak-signal-to-noise ratio (SNR) design point. Increasing the TDC’s full-scale range beyond this point entails increased jitter and, thus, ultimately, a declining SNR. The time-domain peak-SNR happens at a measurement period of around 1/fa, then reduces because of the contribution of low-frequency noise sources, as shown in Figure 4.
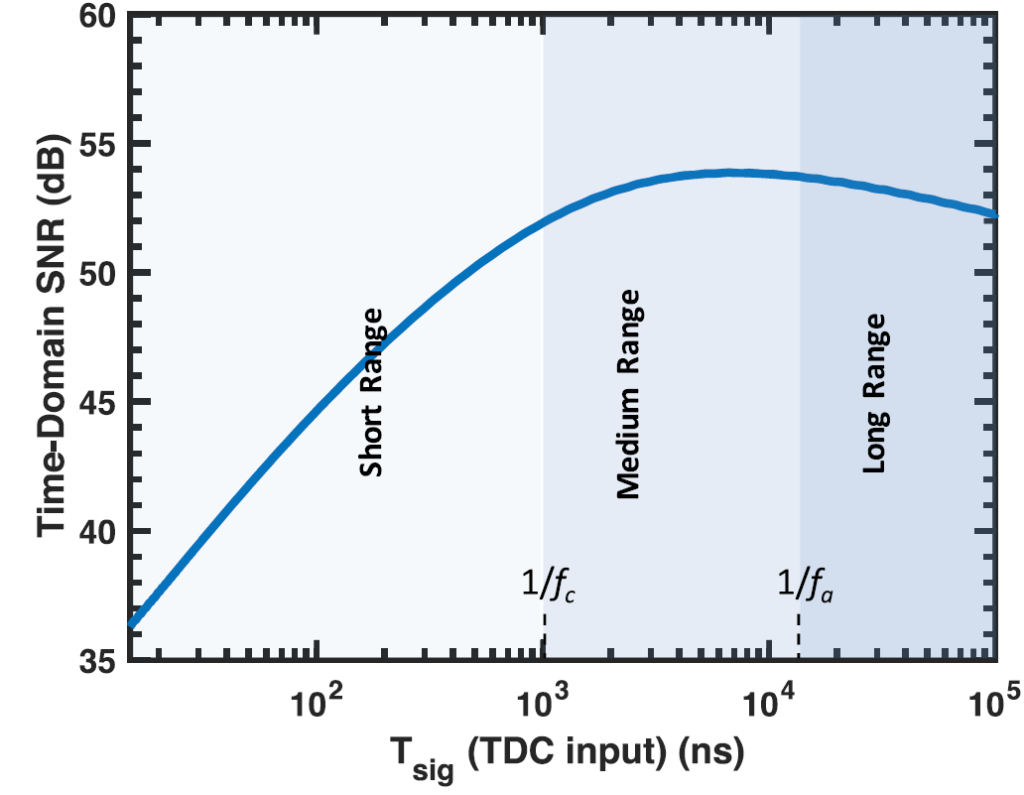
The trend toward larger SPAD arrays and emerging complex TDC-sharing architectures requires much faster simulation methods. We present in our research a simple, fast, and accurate analytical model to address this need [4]. It accounts for dead time effects, which result in missed photon counts through the analysis of inhomogeneous continuous-time Markov chain. The effective received power and photon detection rate are determined, and the corresponding analytical histogram is created. This histogram is the basis for calculating the time of flight and can be used to explore architectural alternatives and accelerate design verification. The outputs of the presented analytical model match those of Monte Carlo simulations and are produced considerably faster. The computation time improvement grows with array sizes, and this enables parametric analysis of the TCSPC system. Figure 5 shows a good match between the analytical histogram and the Monte Carlo result for dead time of 10nsec. It’s worth noting that the simulation time of Monte Carlo analysis strongly depends on the system simulation parameters, such as incoming photon rate and dead time. Simulations are run with MacBook Pro with 2.7 GHz Intel Core i5 and 8GB of memory. For this case, the calculation time is approximately 5000 times faster for the analytical simulation, and this improvement grows with the increase in SPAD array size.
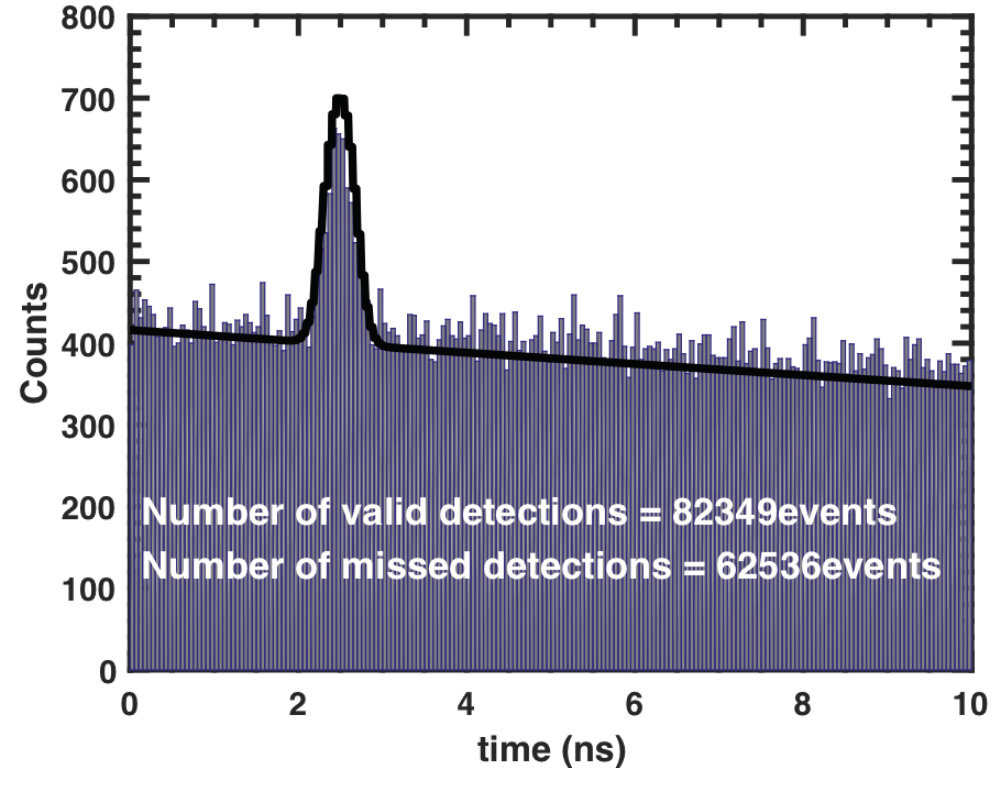
[1] F. Arvani, and A. Chan Carusone “A Reconfigurable 5-Channel Ring-Oscillator-Based TDC for Direct Time-of-Flight 3D Imaging,” IEEE Transactions on Circuits and Systems II: Express Briefs, March 2022. [Open Access on IEEExplore]
[2] F. Arvani, A. Chan Carusone, “TDC Sharing in SPAD-Based Direct Time-of-Flight 3D Imaging Applications,” International Symposium on Circuits and Systems, Sapporo, Japan, May 2019. [On IEEExplore]
[3] F. Arvani and A. Chan Carusone, “Peak-SNR Analysis of CMOS TDCs for SPAD-Based TCSPC 3D Imaging Applications,” IEEE Transactions on Circuits and Systems II: Express Briefs, Oct. 2021. [Open Access on IEEExplore]
[4] F. Arvani, A. Chan Carusone, “Direct Time-of-Flight TCSPC Analytical Modeling Including Dead-Time Effects,” International Symposium on Circuits and Systems, Florence, Italy, May 2018. [On IEEExplore]